How Autonomous Vehicles Can Predict Pedestrians More Precisely
- Virtuaelle reala
- Feb 21, 2019
- 2 min read
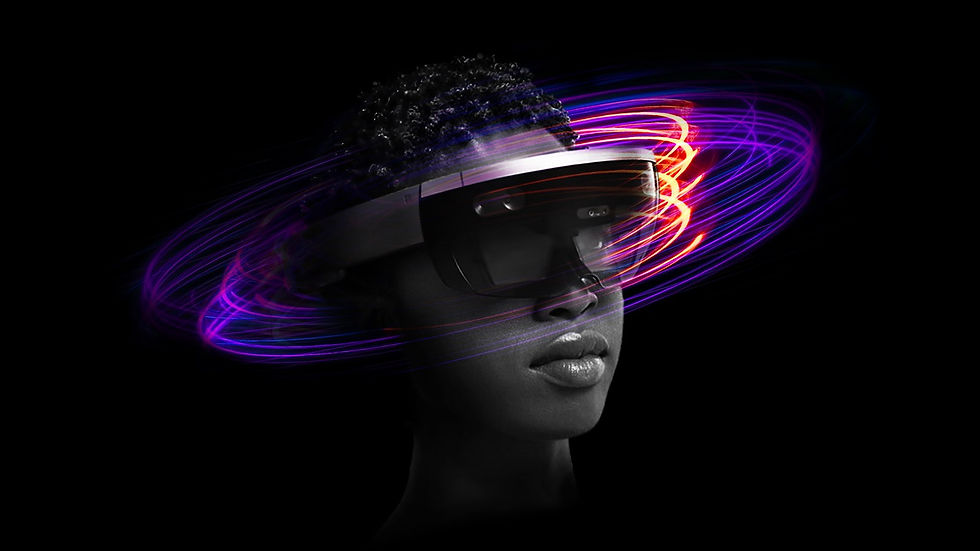
Scientists at the University of Michigan want to teach autonomous vehicles to predict the movements of pedestrians more accurately. Among other things, video clips, 3D simulations and a neural network are used.
Autonomous vehicles, which collect data via cameras, LIDAR and GPS, allow developers to capture video snippets of people in motion and then replicate them in the 3D simulation on the computer. In doing so, they have created a biomechanically inspired, feedback (recurrent) neural network that catalogs human movements.
So it is possible to predict attitudes and location changes of pedestrians within a radius of 45 meters around the vehicle, ie on the order of an urban road intersection.
Previous work in this area has only studied still images, says Ram Vasudevan, a professor of mechanical engineering at the University of Michigan (U-M). But they did not look at how people actually move in three dimensions. However, when autonomous vehicles are in the real world and interact with them, one must be proactive in ensuring that pedestrian and vehicle movements do not get in each other's way.
Developing vehicles with predictive competence requires a network that delves into the tiniest details of human locomotion: the gait rhythm (periodicity), the symmetry of the limbs, and the way feet are set up to influence the stability of walking.
Machine learning, as used for current autonomous technologies, is often based on two-dimensional photos. Finally, a computer with millions of photos of a stop sign will immediately recognize the stop sign in the real world. By using video clips lasting a few seconds, the UM system can now use the first part of the clip for prediction and then verify it in the second part.
Currently, the system is being trained not only to detect the movements of a single object and make predictions, but also to predict where the pedestrian's body will be in the next step, the one after, and so on, says Matthew Johnson-Roberson, a professor at the Department of Naval Architecture and Marine Engineering of UM. With a catchy comparison, Ram Vasudevan describes how the neural network extrapolates.
"When pedestrians play with their cell phones, they know that they are distracted. Their attitude and their gaze draw attention to what they are about and what they might do next. "The new solution helps autonomous vehicles to better understand what is likely to happen in the next moment.
The median deviation of the prediction was 10 cm after one second and less than 80 cm after six seconds. Comparable solutions were up to 7 meters off, as Johnson-Roberson notes.
To limit the variety of predictive capabilities, scientists have considered the limited human physique, such as the highest possible pace or the fact that we can not fly. For the record that was supposed to train the neural network, researchers parked a Level 4 vehicle at various intersections in Ann Arbor. With the cameras and LiDAR, the car was able to record numerous data over many days. These "wilderness" observations were supplemented with conventional housing data obtained in the laboratory. Thus, a system has emerged that raises the bar for the capabilities of autonomous vehicles.
A paper is online in the IEEE Robotics and Automation Letters. It will also appear in the upcoming print edition of the journal. The work was supported by a donation from the Ford Motor Company.
Comments